Michal Segal Rozenhaimer (she/her)
Senior Research Scientist, Assistant Research Director
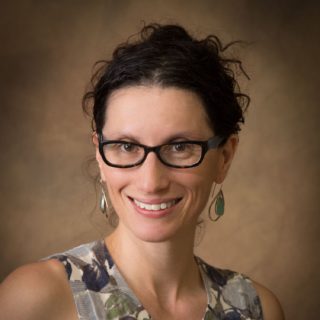
I investigate the link between what is up in the atmosphere and how it affects our climate using measurements, modeling, and big data tools.
Current research
Questions
How can we improve our understanding of the atmospheric processes that are being simulated in climate models so we can gain better insights for the future?
Approach
I use atmospheric measurements (from satellite, airborne, and ground-based platforms), together with big-data and machine learning techniques, to better answer questions about atmospheric process mechanisms, and improve their implementation in global climate models.
Innovations & Discoveries
I developed a machine learning based cloud mask algorithm for WV-2 satellite (Segal Rozenhaimer et al., 2020) that is now being applied and extended for the Venus satellite to include cloud masking over deserts.
Segal Rozenhaimer Michal, Alan Li, Kamalika Das and Ved Chirayath, (2020), Cloud Detection Algorithm for Multi-Modal Satellite Imagery using Convolutional Neural-Networks (CNN), Remote Sensing of Environment, Remote Sensing of Environment 237 (2020) 111446
Technical Description
My work combines going to the field and taking measurements, either with ground-based remote sensing instruments that can measure aerosol amount and properties, or by flying with various instruments on airplanes. I measure the properties of aerosols, clouds and gases either remotely (optically), or in-situ, which means drawing air from outside of the plane and measuring the particles in the airflow. Most of the measurements require lots of post-processing and analysis work so that the actual properties we are interested in measuring will be available (to us or to the scientific community). For example, in remote sensing (optical) measurements, we need to process the data using mathematical inverse modeling methods that can extract (retrieve) the quantities we are looking for. A large portion of my work includes the development of such retrieval methods from various instruments using radiative transfer simulations and neural-networks. When we sample air from outside the plane, we can run the analysis in the plane (online) or take our samples to the lab and analyze them there (offline).
The second major part of my work involves tying these measurements to process understanding in order to improve upon climate models. As climate models cannot resolve many of the aerosol or cloud processes, they use schemes that are called parameterizations. By utilizing real measurements we can learn more about certain processes and try to model/parameterize them better in models, which can help climate predictions.
Publications
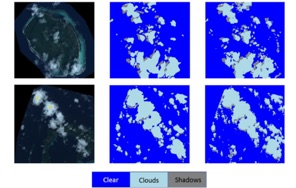
Cloud and cloud shadow masks developed for WV-2 satellite based on CNN (Segal-Rozenhaimer et al., 2020) - NeMO-Net Project.
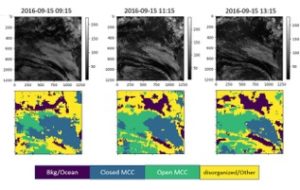
Time-series of SEVIRI satellite images over the South-East Atlantic Ocean, with their corresponding cloud type masks - ACMAP project.
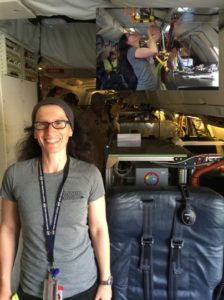
At work!
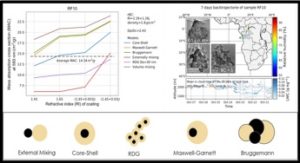
ORACLES Research Flight 010 (Oct-17-2018) trajectory (top right), including time in cloud, with analyzed TEM imagery of some BC (black carbon) mixed particles, and their calculated MACs for various optical mixing models - DOE-ASR project.
Education
PhD from the Technion-Israel Institute of Technology
NASA Post-doctoral Fellow
NASA Post-doctoral Fellow
Get in touch: segalrozenhaimer (at) baeri.org
Current Projects
NASA AIST — NeMO-Net - The Neural Multi-Modal Observation & Training Network for Global Coral Reef
Machine learning is a fast-paced emerging field that is utilized frequently in our daily lives, including google search engines, self-driving cars, natural language processing and much more. Machine learning methods excel at discovering patterns and recognizing similarities within a massively multivariate data system or one composed of thousands of variables, which is a great fit for hyperspectral and multi-spectral remote-sensing satellite imagery.
We work on the development of machine learning based algorithms to retrieve aerosol and cloud properties from remote sensing measurements: see our recent paper, which is a part of the NASA NeMO-Net project: http://nemonet.info/
Segal-Rozenhaimer, M., et al. (2020), Cloud detection algorithm for multi-modal satellite imagery using convolutional neural-networks (CNN), Remote Sensing of Environment, 237, 111446, doi:10.1016/j.rse.2019.111446.
We work on the development of machine learning based algorithms to retrieve aerosol and cloud properties from remote sensing measurements: see our recent paper, which is a part of the NASA NeMO-Net project: http://nemonet.info/
Segal-Rozenhaimer, M., et al. (2020), Cloud detection algorithm for multi-modal satellite imagery using convolutional neural-networks (CNN), Remote Sensing of Environment, 237, 111446, doi:10.1016/j.rse.2019.111446.
NASA ACMAP (Atmospheric Composition and Modeling)
Improving our understanding on the Link between Aerosols, Marine Stratocumulus Clouds and Radiative Effects: Combining Novel Cloud Classification Approach with Satellites, Airborne Observations and Climate Models
We are developing an algorithm to detect marine stratocumulus cloud types (Marine Cellular convection) from geostationary satellites to better understand their dynamics and formation mechanisms (NASA ACMAP funded project)
We are developing an algorithm to detect marine stratocumulus cloud types (Marine Cellular convection) from geostationary satellites to better understand their dynamics and formation mechanisms (NASA ACMAP funded project)
DOE-ASR — Assessing the link between aerosol mixing state, structure and composition and their optical properties: Ascension Island as a testbed for the South-East Atlantic aerosol regimes
Working to understand how aerosol mixing state, chemical composition, morphology, and microstructure affect bulk optical properties such as the single-scattering albedo (SSA), and how they affect the sign of the net top-of-atmosphere radiative forcing. In this DOE-ASR funded project we investigate how aging time in the atmosphere and cloud processing time and extent might affect the mixing state of the biomass-burning aerosol particles that were sampled in ORACLES, as well as other campaigns including the UK-Met Office CLARIFY and LASIC, and how these affect their ability to absorb visible light.
About Michal
Where are you from, and what were you doing before BAERI?
I grew up in Israel and currently go back and forth between the US (West Coast) and Israel. Before coming to BAERI I was a post-doc fellow (NPP program) at the NASA Ames Research Center.
What’s one job-related thing that you love talking about with other people?
Planning new ground-based and airborne missions.
How about a non-job related thing?
Nature!
We are a community of researchers, engineers,
explorers, writers, and more. Our shared belief
in the power of science inspires our work to
better understand our planet, and the universe.
explorers, writers, and more. Our shared belief
in the power of science inspires our work to
better understand our planet, and the universe.